Announcing Pienso NLQ and PromptFactory
NLQ transforms AI search into a structured, interactive data exploration process, while PromptFactory removes the complexity of prompt and flow engineering.
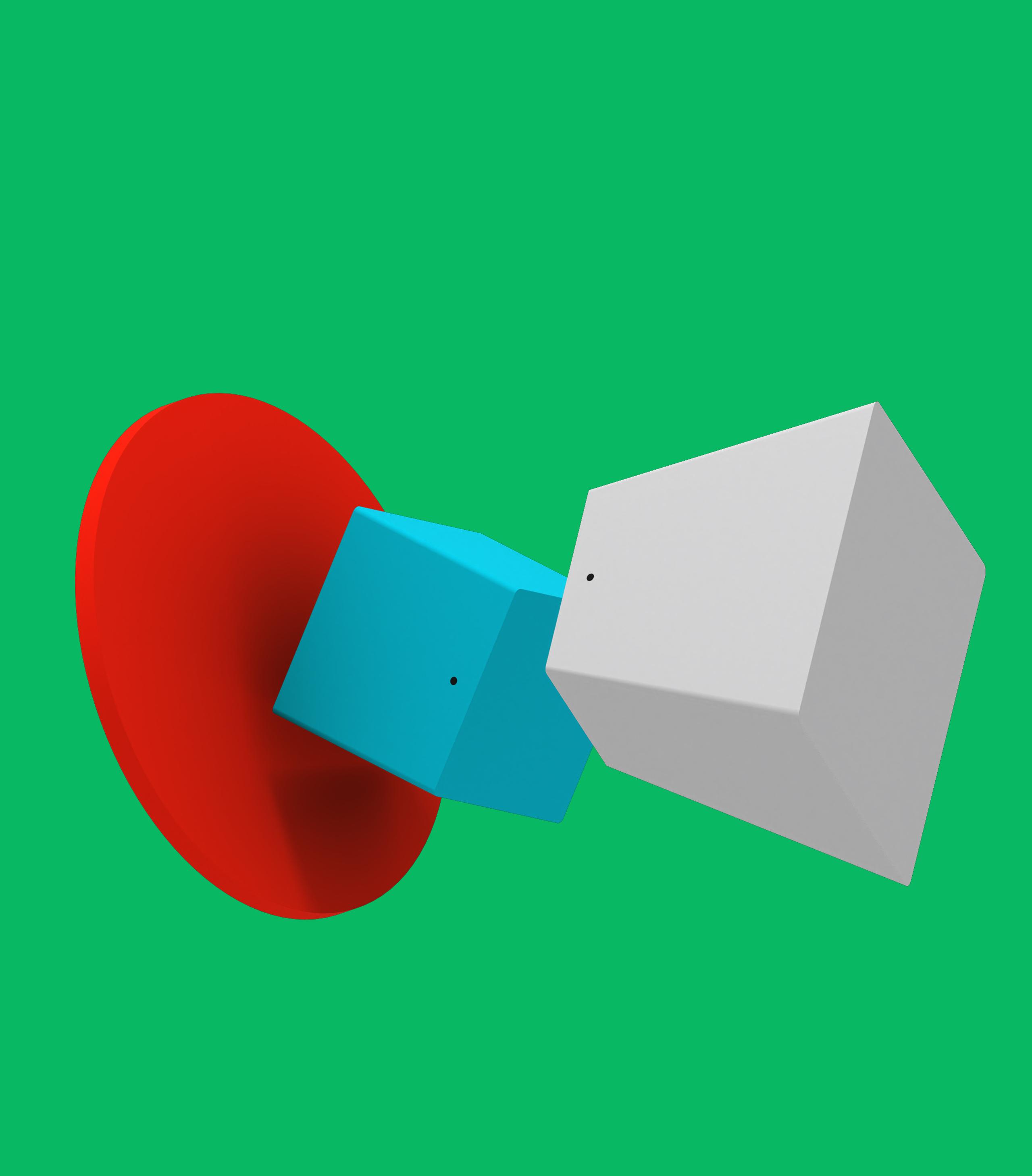
We’re thrilled to introduce two new products from Pienso designed to revolutionize how you interact with your data: Pienso NLQ and Pienso PromptFactory. Both of these tools are currently in beta, but they’ve already received a warm welcome from our early users. These innovations make AI more accessible, especially for subject matter experts who might not have a background in coding. Here’s what you need to know about these exciting new offerings.
Pienso NLQ
Pienso NLQ (Natural Language Query) combines search capabilities with auto-regressive models, offering a more structured and interactive way to explore your data. Unlike typical AI search systems (known as retrieval-augmented generation, or RAG systems) that only provide final answers, NLQ engages you in each step of the search process. This means you can ask questions and explore insights with structured templates and step-by-step sequences, giving you better control and understanding of the search process.
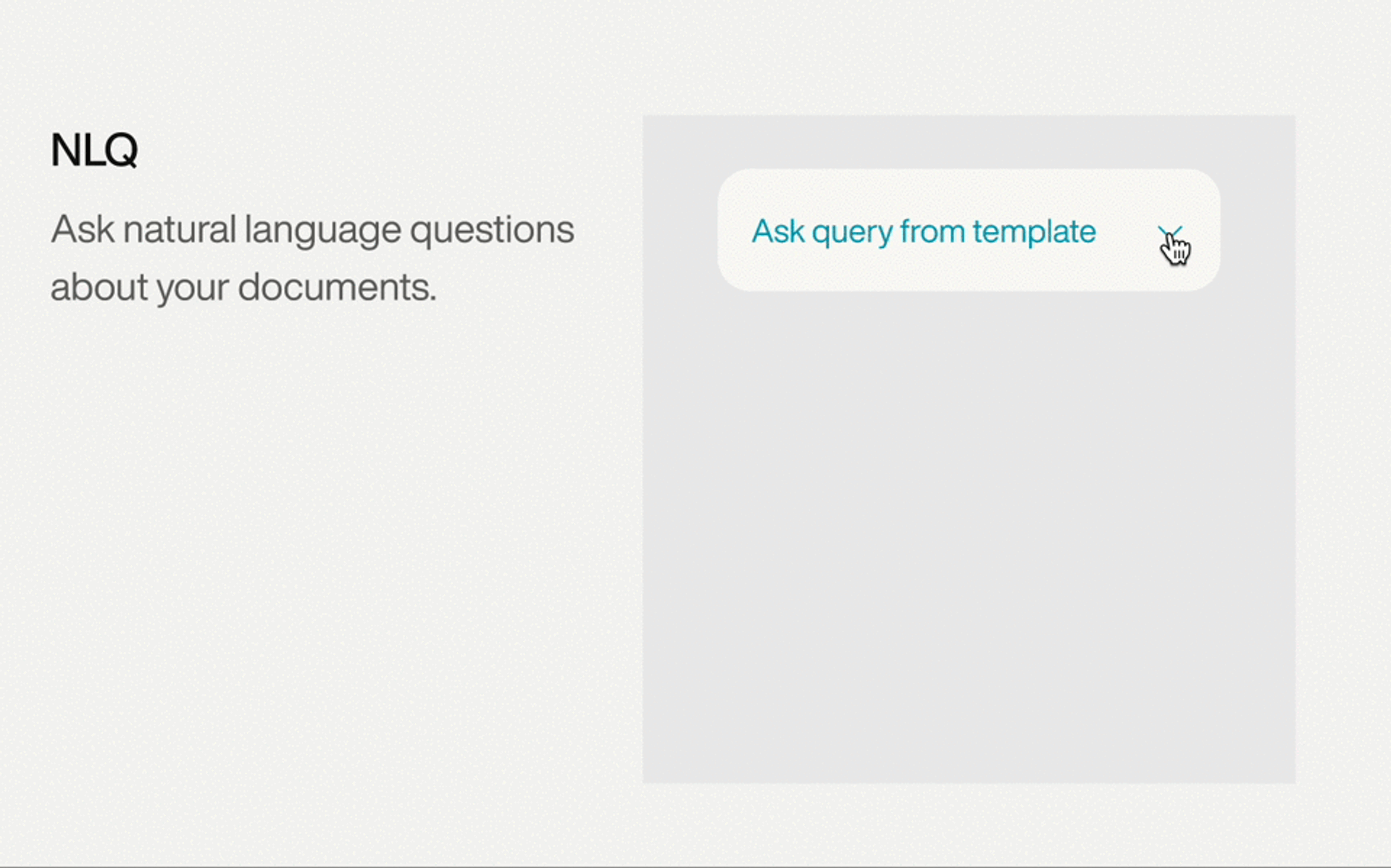
Key Features of Pienso NLQ
- Structured Templates: Our templates help you ask clear and precise questions.
- Interactive Design: Engage directly with your data and refine your queries to uncover deeper insights.
- User-Friendly Interface: Designed to be accessible and intuitive, even if you don’t have a technical background.
- Balanced Approach: We combine search and auto-regressive models with a structured yet interactive user interface to create a more nuanced and reliable tool compared to traditional RAG systems.
Since the 1990s the AI community has debated the effectiveness of agents versus direct manipulation. Drawing from our experiences and research at MIT, we’ve taken a balanced approach— prioritizing user-friendly design and robust computational steps. This addresses common frustrations users have with traditional RAG systems, such as irrelevant or incorrect answers and lack of transparency.
Pienso PromptFactory
Pienso PromptFactory simplifies the creation, evaluation, and deployment of auto-regressive models for tasks like classification, extraction, and summarization. This tool removes the complexities of prompt and flow engineering, allowing you to extract insights from your data without needing to write code.
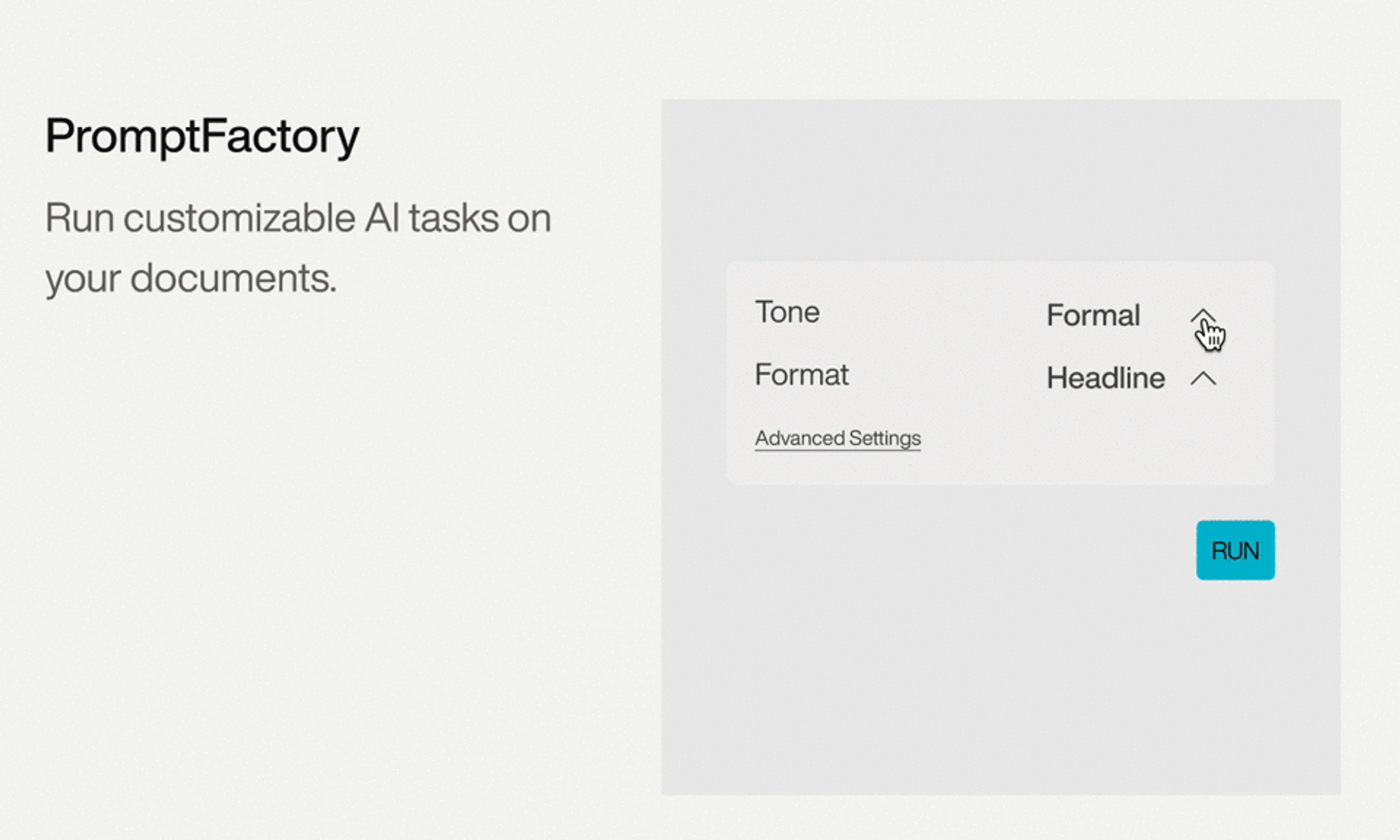
Key Features of Pienso PromptFactory
- Ready-to-Use Templates: Pre-designed templates make it easy to create complex prompts.
- Efficient Pipelines: Streamline the process of deploying prompts into production with robust, behind-the-scenes pipelines.
- User Empowerment: Retain control and flexibility to apply your subject-specific knowledge.
- No Coding Needed: Accessible to a broad audience, regardless of technical expertise.
PromptFactory is ideal for enterprises implementing AI for tasks like automating data classification, extracting key information, or generating summaries. It simplifies the process and ensures quick deployment of effective AI solutions.
Although both Pienso NLQ and Pienso PromptFactory are still in beta, initial feedback from our customers has been overwhelmingly positive. This enthusiasm highlights the practical value and potential these tools bring to real-world use-cases, as well as our commitment to user-friendly design and functionality.
Some references you might be interested in reading:
- Shneiderman, B. (1997). Direct manipulation for comprehensible, predictable, and controllable user interfaces. Proceedings of the 2nd International Conference on Intelligent User Interfaces.
- Maes, P. (1994). Agents that reduce work and information overload. Communications of the ACM, 37(7), 30-40.
- Lewis, M., Liu, Y., Goyal, N., Ghazvininejad, M., Mohamed, A., Levy, O., ... & Zettlemoyer, L. (2020). Retrieval-augmented generation for knowledge-intensive NLP tasks. Advances in Neural Information Processing Systems, 33, 9459-9474.
- Karpukhin, V., Oguz, B., Min, S., Lewis, P., Wu, L., Edunov, S., ... & Yih, W. (2020). Dense passage retrieval for open-domain question answering. arXiv preprint arXiv:2004.04906.