Thinking About Compute Power Responsibly
Pienso's dedication to proactive, well-informed steps to address carbon emissions.
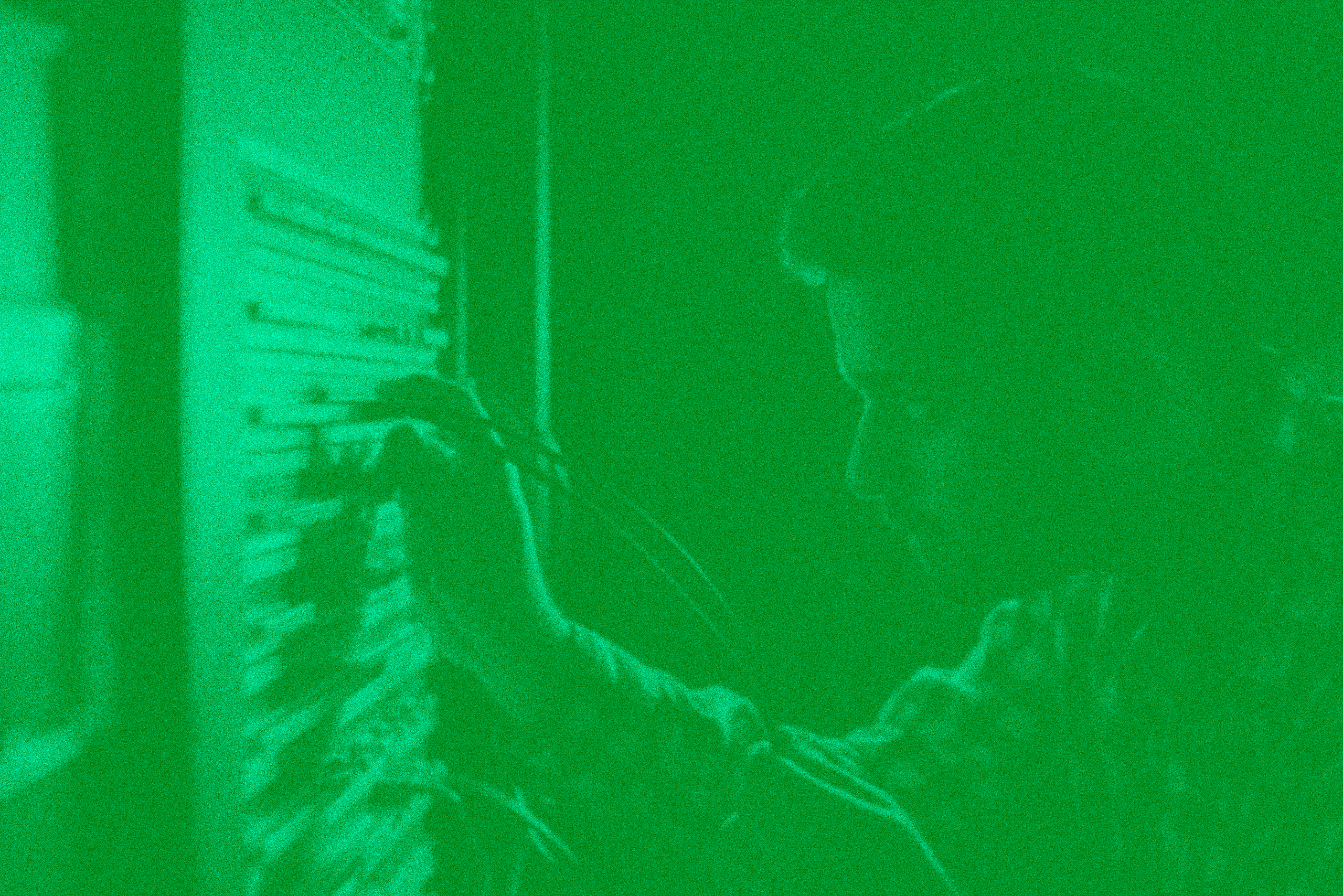
A growing issue in the rapidly evolving field of large language models (LLMs) is how compute costs may affect the environment. As LLMs become increasingly ingrained in our daily lives and enterprises, we must confront the significant energy requirements — and corresponding carbon emissions — that these technologies demand. At Pienso, we're dedicated to not only recognizing these difficulties but also taking proactive, well-informed steps to address them.
Due in large part to their operational and training requirements in data centers, very large language models (LLMs) such as GPT-4 come with considerable computational requirements and environmental expenses. These models necessitate large computational resources, such as powerful GPUs and a lot of electricity, which add significantly to energy consumption and carbon emissions.
These models are frequently produced and run in data centers, which are estimated to contribute between 1% and 1.5% of the world's electricity consumption and 0.6% of its carbon emissions. The particular environmental effect is contingent upon the energy sources employed. Compared to data centers that use fossil fuels, ones fueled by renewable energy sources can drastically cut carbon emissions1.
It takes tens of thousands of high-performance CPUs and a significant amount of energy to train a huge model such as GPT-4o. It’s been estimated that the training of GPT-3 alone consumed around 1.3 gigawatt-hours of energy, or the annual energy usage of about 120 ordinary U.S. houses. To handle the heat produced by high-density computers, data centers with sophisticated cooling systems are required as part of the infrastructure needed for these computations. More effective liquid cooling technologies are gradually replacing conventional air-cooling systems because they lower operating costs and energy usage2.
There are measures in place to lessen the impact of artificial intelligence on the environment. These measures center on maximizing the efficiency of hardware, algorithms, and the time and place of model training in relation to the availability of low-carbon energy. In order to lower computing needs, some strategies may entail training more compact, specialized models for certain tasks3. At Pienso, we think that a garden of more compact but efficient smaller models may save money and the environment while also yielding a significant return on investment.
Whether AI compute is done on private data centers or public clouds can have a substantial impact on the cost. Operating their own data centers may be more cost-effective for large enterprises with complex needs, but doing so necessitates a considerable upfront infrastructure investment4 . All things considered, the drive towards more environmentally friendly AI practices combines cutting-edge hardware with energy-efficient data centers and more astute operational techniques to strike a balance between computational demands and environmental obligations.
I want to highlight a few important areas that we are focusing on to ensure that our computing strategy is as responsible as feasible.
Cloud Deployment Strategy
We work with Google Cloud Platform to strategically place our infrastructure in areas that have been determined to have minimal carbon emissions. We support Google Cloud’s goal5 to match their energy consumption effectively all the time and everywhere with carbon free energy (CFE) by 2030. A great majority of our cloud infrastructure runs in locations where the average percentage of carbon free energy consumed on an hourly basis is over 90 percent6. We intentionally target infrastructure deployment in locations with the highest possible Google CFE%7. Simply put, running Pienso in these data centers drastically lowers our carbon impact.
We’re also trialing Google TPUs for our workloads, given that we’re already on Kubernetes, to lower our overall energy use on Google Cloud. Efficient hardware accelerators like TPUs8 help us drive consumption down. It’s simply not enough to seek the lowest possible carbon footprint through energy generation alone.
Hardware that uses less energy
We work with Graphcore to use their Intelligent Processing Units (IPUs), which are engineered to be more efficient than competing hardware accelerators in terms of performance per watt. As shown by multiple analyses9, this technology allows us to retain high performance levels while drastically reducing our energy use. Hardware accelerator diversity lets us deliver strategically optimized implementations. For Pienso on Graphcore, we partner with Gcore for virtual vPOD10 cloud deployments and Dell for on premise bare metal deployments using IPU-POD16 Direct Attach systems11.
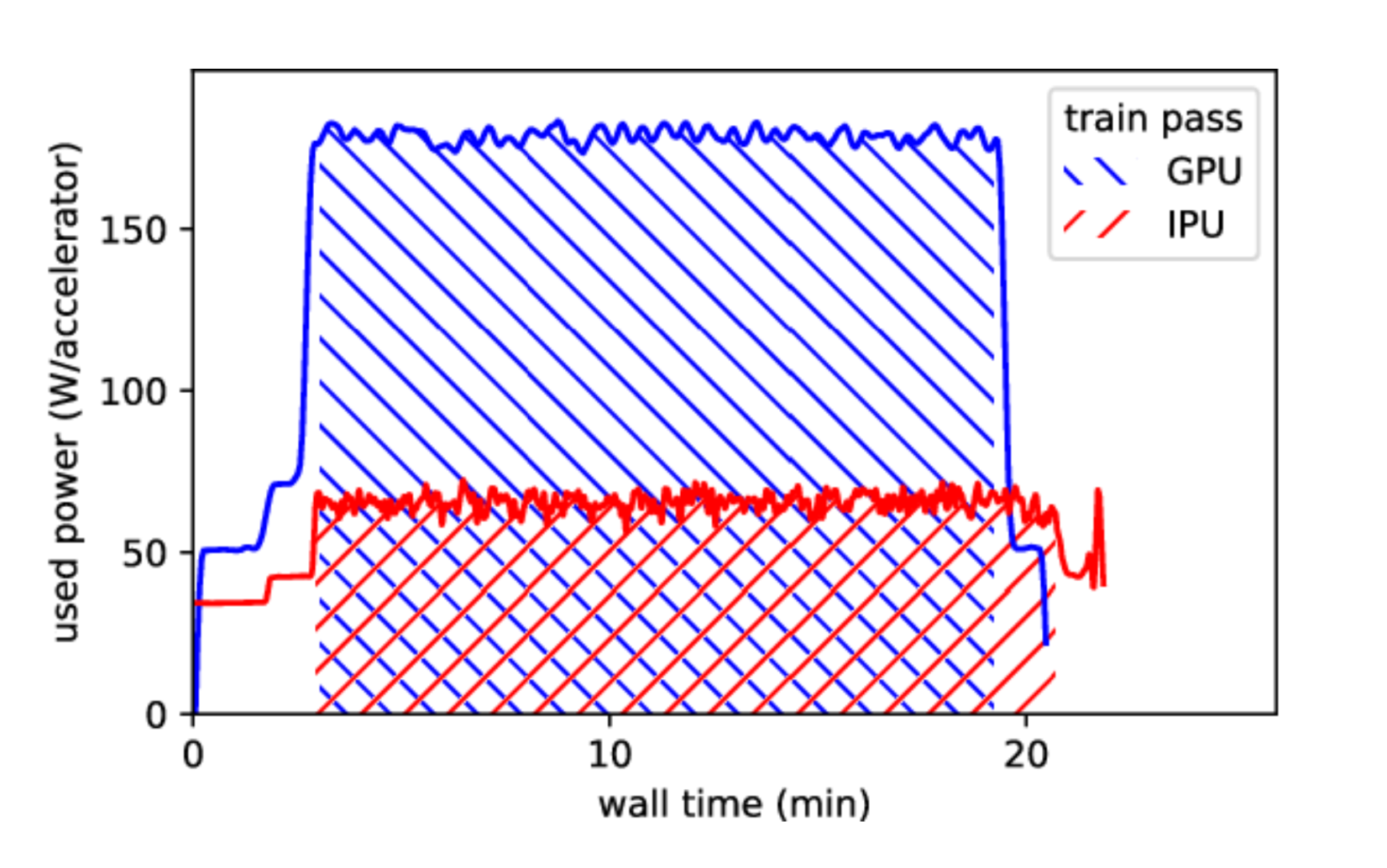
Figure from "Time-series ML-regression on Graphcore IPU-M2000 and Nvidia A100" shows power usage for 16-accelerator training jobs on Nvidia (blue) and Graphcore (red). Although Nvidia A100 GPUs train 15% faster, Graphcore IPU-M2000 IPUs use 2.5 times less total energy to complete the same work.
Optimized On-Premise Hardware
We also use cutting-edge hardware that consumes less power and takes up less space than traditional strategies thanks to our collaboration with Liqid. Liqid’s SmartStack technology dramatically increases the GPU density per attached bare metal host. Furthermore, Liqid’s composability allows us to seamlessly introduce various GPUs in a hot-swappable manner to hosts that otherwise would not be able to accommodate them. This is a sustainable hardware approach that lets us quickly take advantage of the latest LLMs and hardware accelerators with extremely low capital or infrastructure reinvestment. Reducing the overall environmental effect of our physical infrastructure depends on this efficiency.
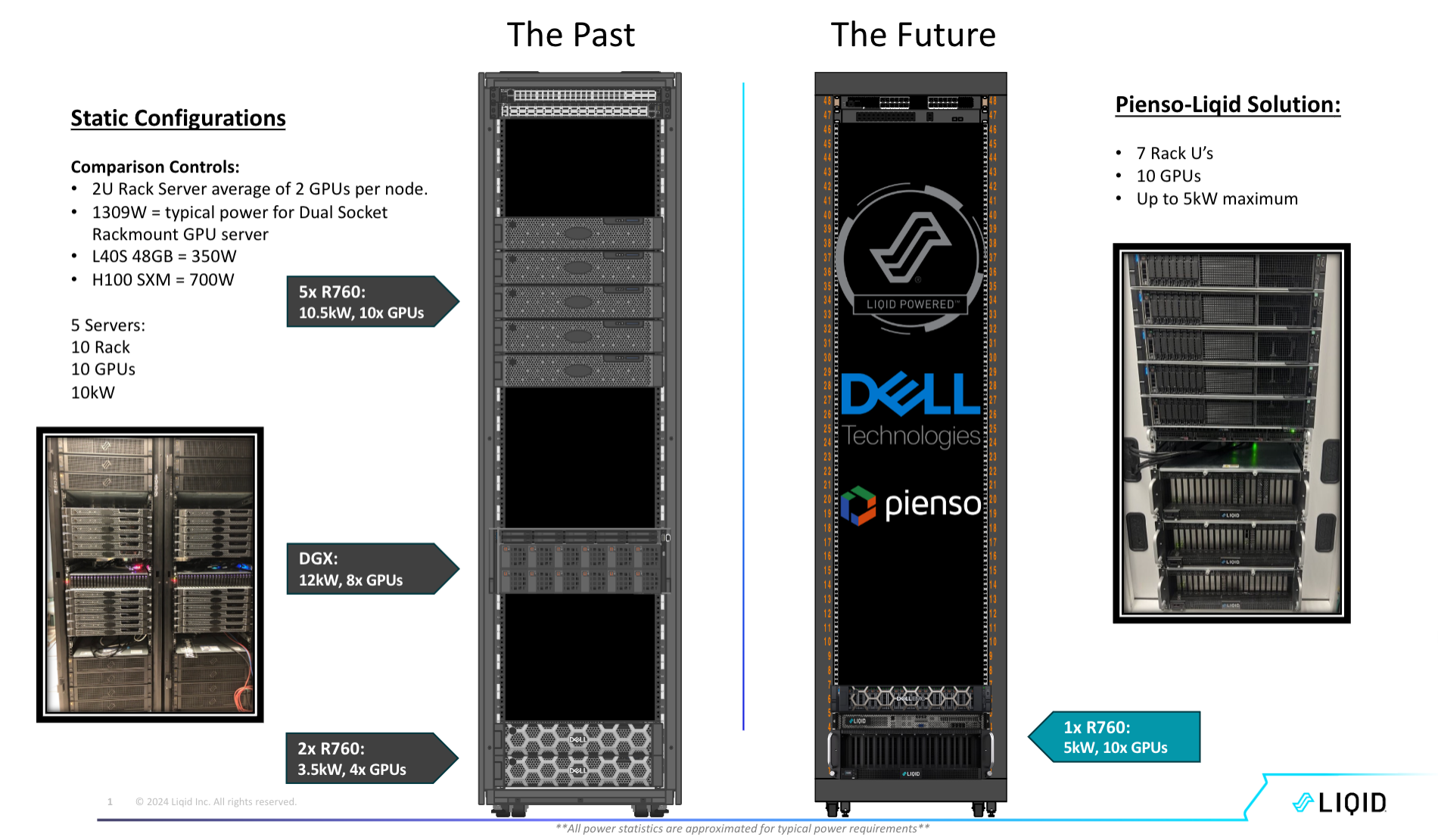
Even while efficiency is our goal, I think we should recognize that technical advancement can initially come with scope for optimization. We keep looking for collaborations and innovations that share our principles, with an emphasis on sustainability and moral responsibility. Our goal is to improve energy consumption by reducing superfluous bloat and optimizing the LLM stack for effective fine-tuning and inference. This strategy fits with our objective of being as responsible and efficient as feasible in addition to assisting with the management of our carbon impact. I'd like to assume that ethics and accountability—rather than merely market expectations or regulatory compliance—are what motivate us to be committed to sustainable AI. I think it's our duty to set an example for others, showing that environmental consciousness and technical growth are compatible.